Introduction of AI for defect Classification of precision equipment parts
At ARAYA, we use image recognition technology based on deep learning to solve a variety of business issues for our customers.
Here, we introduce a case where AI was introduced to classify defects in the manufacturing stage of precision equipment parts.
CHALLENGES BEFORE THE INTRODUCTION OF AI
In the manufacturing process of the client company, defects such as scratches and impurities on the surface of products were occurring at a certain rate. In order to identify the cause of the defects in order to improve the yield rate, the inspectors visually categorized the images taken of the defects and linked them to the improvement of the manufacturing process.
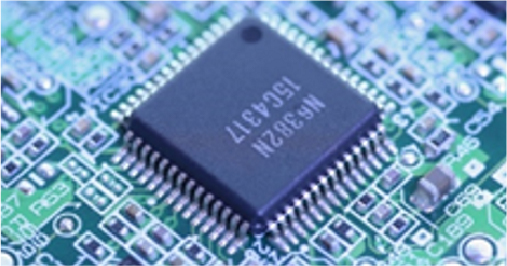
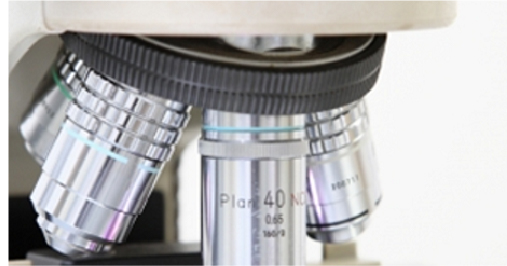
-Then, you had the following challenges.
1. Time consuming defect classification
Defects need to be classified according to their number, position and size. On the other hand, since defects are very minute in size and diverse in type, it is very difficult for the inspector to classify them visually, and a lot of time is required for the work.
2. Variation in the results of defect classification
As mentioned above, visual inspection is difficult, so the results of defect classification were inconsistent. This made it difficult to provide accurate feedback to the manufacturing process.

EFFECTS AFTER AI IMPLEMENTATION
IN ORDER TO SOLVE THE ABOVE ISSUES, WE INTRODUCED AN AI ALGORITHM THAT CLASSIFIES IMAGES OF A WIDE VARIETY OF DEFECTS BY EVENT, WHICH SIGNIFICANTLY IMPROVED THE ACCURACY OF DEFECT CLASSIFICATION AND IMPROVED THE EFFICIENCY OF THE INSPECTION PROCESS.
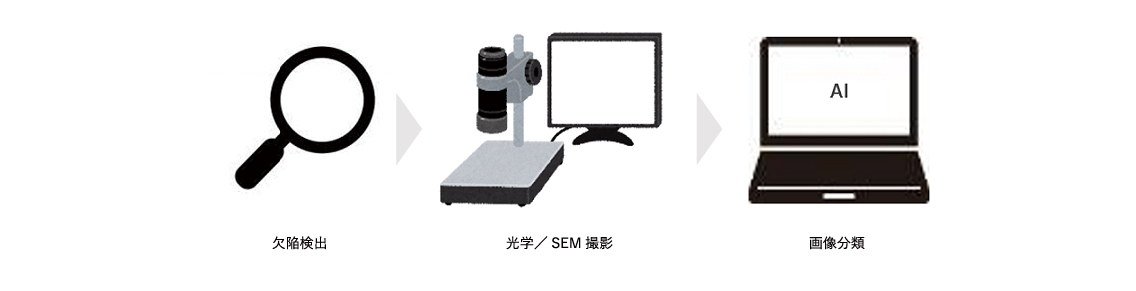
-Specifically, the following effects were achieved.
1. Improved inspection efficiency by shortening the time required
BY USING AI TO PERFORM MOST OF THE VISUAL DEFECT CLASSIFICATION WORK, WE WERE ABLE TO SHORTEN THE TIME REQUIRED FOR CLASSIFICATION WORK. THE INSPECTOR ONLY HAS TO CLASSIFY A SMALL NUMBER OF DEFECTS THAT THE AI COULD NOT CLASSIFY, LEADING TO AN IMPROVEMENT IN INSPECTION EFFICIENCY.
2. Significantly improved defect classification accuracy and manufacturing process
WITH THE INTRODUCTION OF AI, THE DEFECT CLASSIFICATION ACCURACY OF THE CAPTURED IMAGES HAS BEEN GREATLY IMPROVED WITH A CORRECT CLASSIFICATION RATE OF 96.47%. THIS HAS MADE IT POSSIBLE TO PROVIDE ACCURATE FEEDBACK FOR MANUFACTURING PROCESS IMPROVEMENT.
POINTS TO CONSIDER WHEN DEVELOPING AI
[Point 1] Sorting of defects by image classification system
THE AI IMAGE CLASSIFICATION SYSTEM (CNN MODEL) DETECTS DEFECTS IN PIXEL UNITS FROM IMAGES OF THE PRODUCT SURFACE TAKEN BY SEM, AND AUTOMATICALLY CLASSIFIES THE TYPE OF DEFECT. THE INSPECTOR NOW ONLY NEEDS TO VISUALLY CLASSIFY A SMALL NUMBER OF IMAGES WHERE THE ACCURACY OF THE AI JUDGMENT IS LOW.
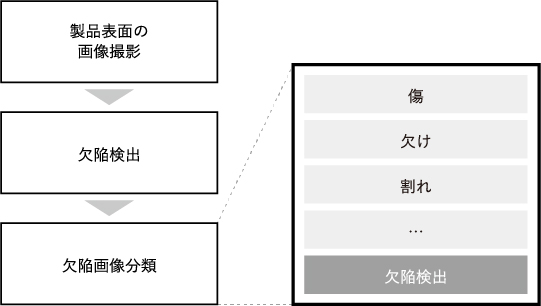
2] Visualization and mapping of classification results to improve inspection efficiency
By integrating defect location information and classification results, we were able to visualize what defects occur where. This has greatly improved the efficiency of classification inspection.
Integration of location information and defect classification results
Identify and map the location of partial images of only defects in the overall product image.
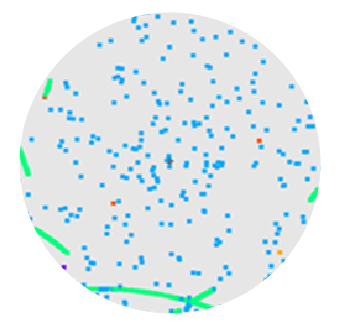