REALISATION OF AGI WITH ‘CONSCIOUSNESS’ CAPABILITIES(2):
INFORMATION GENERATION THEORY – ‘Imaging’ Imaginary Situations
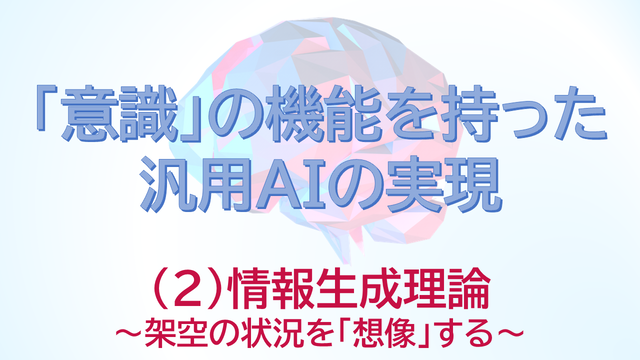
What kind of "consciousness" does a human being have - at ARAYA, we are continuing our research to answer this question. By creating an artificial equivalent of consciousness (AI), we are trying to get to the bottom of it and realize a "general-purpose AI".
In a four-part series, Ryota Kanai, President of the company, will explain how he plans to approach the project in concrete terms.
Realization of general-purpose AI with the function of "consciousness" (1): Overview and three hypotheses
Realization of general-purpose AI with the function of "consciousness" (2): Information generation theory - "imagining" an imaginary situation (this article )
Realisation of general-purpose AI with 'consciousness' functions (3): Global workspace theory - bridging information between brain modules
Realization of general-purpose AI with the function of "consciousness" (4): Meta-representation theory of qualia - Being able to cope with new tasks
Information generation theory: the ability to 'imagine' imaginary situations
In the previous article, we introduced three hypotheses for realizing a general-purpose AI with the function of "consciousness". In this article, we introduce the information generation theory (*1).
1: R. Kanai et al., "Information generation as a functional basis of consciousness," Neuroscience of Consciousness, Vol. 2019, Issue 1, 2019.
The function of consciousness, as envisaged by this theory, is to create information in the mind that is different (counterfactual-virtual) from the environment of the here and now. Roughly speaking, it corresponds to the ability to "imagine" a situation different from the present one. Thanks to this function of consciousness, we can simulate things in advance, for example, before we take action or make long-term plans. We call this 'counterfactual simulation'.
The core element of this theory is the assumption that there is a model of the real world in the brain that can be used for simulation. We believe that we acquire models of the world around us through our sensory organs and motor interactions, and that we use these models to predict what will change when we act. So the first step in giving ai the capabilities of consciousness starts with building the ability to model the world.
GQN TECHNOLOGY FOR A COMPACT REPRESENTATION OF THE WORLD
The key to modelling the world is to make it as compact as possible. If the state of the world were to be stored and reconstructed as a vast amount of (high dimensional) information, such as images or 3D models, the limited resources of the brain would be used up very quickly. It is important to capture the essential features of the world and to reproduce its behaviour with as little information as possible.
Recent advances in deep learning technology have given us clues to the means to meet this requirement. One technology we are interested in is the Generative Query Network (GQN ) (*2), published in 2018 by DeepMind, an affiliate of Google, which makes it possible to describe the state of a 3D space in a CG-represented indoor environment, with objects of various shapes and colours, using only 256 (256 This is a much smaller amount of information than when using 3D models or images to represent the world.
*2: S. M. A. Eslami et al., "Neural scene representation and rendering," Science, Vol. 360, 2018.
What's great about GQN is that even if you've never seen a place before, if you know how it looks from a few different angles, you can make up for all the rest. By simply "photographing" a 3D CG room with several objects, such as a sphere or a cone, from two or three different viewpoints, and then inputting the images into GQN, it can output images seen from every position in the room. In fact, many AI researchers have been amazed by the images that GQN draws as it smoothly changes viewpoints, as if it is moving around the room, and they look exactly the same as the 'correct' images. In other words, GQN can "imagine" the parts of the scene that are hidden in the shadows in the given image.
The secret behind the detachment demonstrated by gqn lies in its prior training on millions of images. By preparing and training a large number of CG scenes with different looks for walls and floors, and different colours and shapes for objects, GQN was able to extract the common features of the scenes and put all the information about the 3D environment in the images into 256 variables.
Moreover, GQN has learned the distribution of possible values for each variable (probability density function) and is able to link this distribution to the viewpoint from which each image was "taken". This ability means that given a few sample images and a viewpoint position, GQN can produce images that are probabilistically likely to be seen from that position.
MECHANISM OF THE VISUAL CORTEX OF THE BRAIN MATCHES THAT OF THE VAE
DeepMind used a type of deep learning technology called VAE (Variational AutoEncoder ) (*3) to learn GQN. We have high hopes for VAE as a candidate technology for building models of the world, and we assume that learning mechanisms similar to VAE are built into the human brain. These mechanisms in the brain are thought to be involved in the development of consciousness.
3: D. P. Kingma et al., Auto-Encoding Variational Bayes, https://arxiv.org/abs/1312.6114
VAE is a method based on AE (AutoEncoder), a deep learning technique used for anomaly detection. When an image is input, the encoder compresses the image data (extracting the values of the variables) and the decoder restores the original image. The variables output by the encoder are called latent variables and can be regarded as essential information for the representation of the image.
To be more precise, while AE is a method to obtain the latent variables of the original image, VAE is a method to estimate the mean and variance of the probability distribution followed by the latent variables of the image. Incidentally, GQN uses Conditional VAE, which is a development of VAE, and it is trained to estimate the probability distribution of the latent variable of the original image, with the addition of several sample images of the current environment and the viewpoint position of the original image.
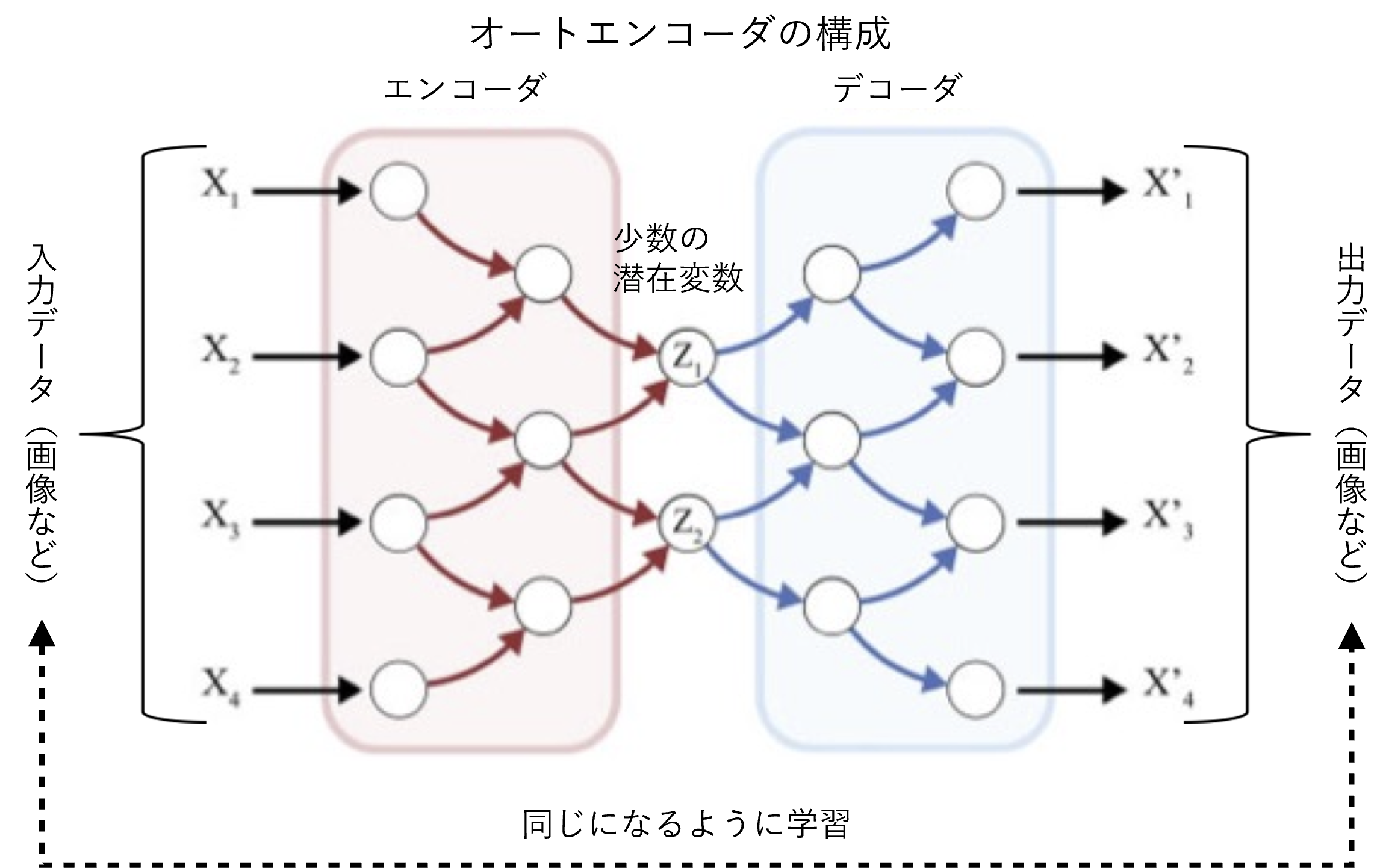
A mechanism similar to the encoder-decoder relationship in AE has been proposed as a theory for the visual cortex of the brain. The theory is called " predicative coding". The visual cortex of the brain has a multi-layered hierarchy, from the lower regions that handle the 'raw' information from the eyes to the higher regions that are responsible for more sophisticated processing such as object recognition. The higher up the hierarchy you go, the more redundancies are removed from the huge amount of information in the image, and the less essential information is left. Predictive coding (*4) is a mechanism for extracting more accurate information from such a series of processes.
4: R. P. N. Rao et al., "Predictive coding in the visual cortex: a functional interpretation of some extra-classical receptive-field effects," Nature Neuroscience, vol. 2, no. 1, January1999.
Between each layer of the visual cortex, there are two paths: (1) a pathway that conveys signals from higher to lower levels, and (2) the opposite pathway that goes from lower to higher levels. In the theory of predicative coding, the higher level region predicts the image information being processed in the lower level region based on the information at hand, and transmits it sequentially through the pathway (1). On the other hand, the lower region sends a signal corresponding to the difference (error) between the received prediction and the actual information to the upper region via the path (2). The higher-level region receives this signal and corrects the error, thus making a more accurate prediction possible. By repeating this process of prediction and correction, the brain is able to perceive the world correctly, which is the essence of Predictive Coding.
We see the role of the (1) higher-to-lower pathway in predicative coding as being consistent with the decoder in AE, and the role of the (2) lower-to-higher pathway as being consistent with the encoder in AE.
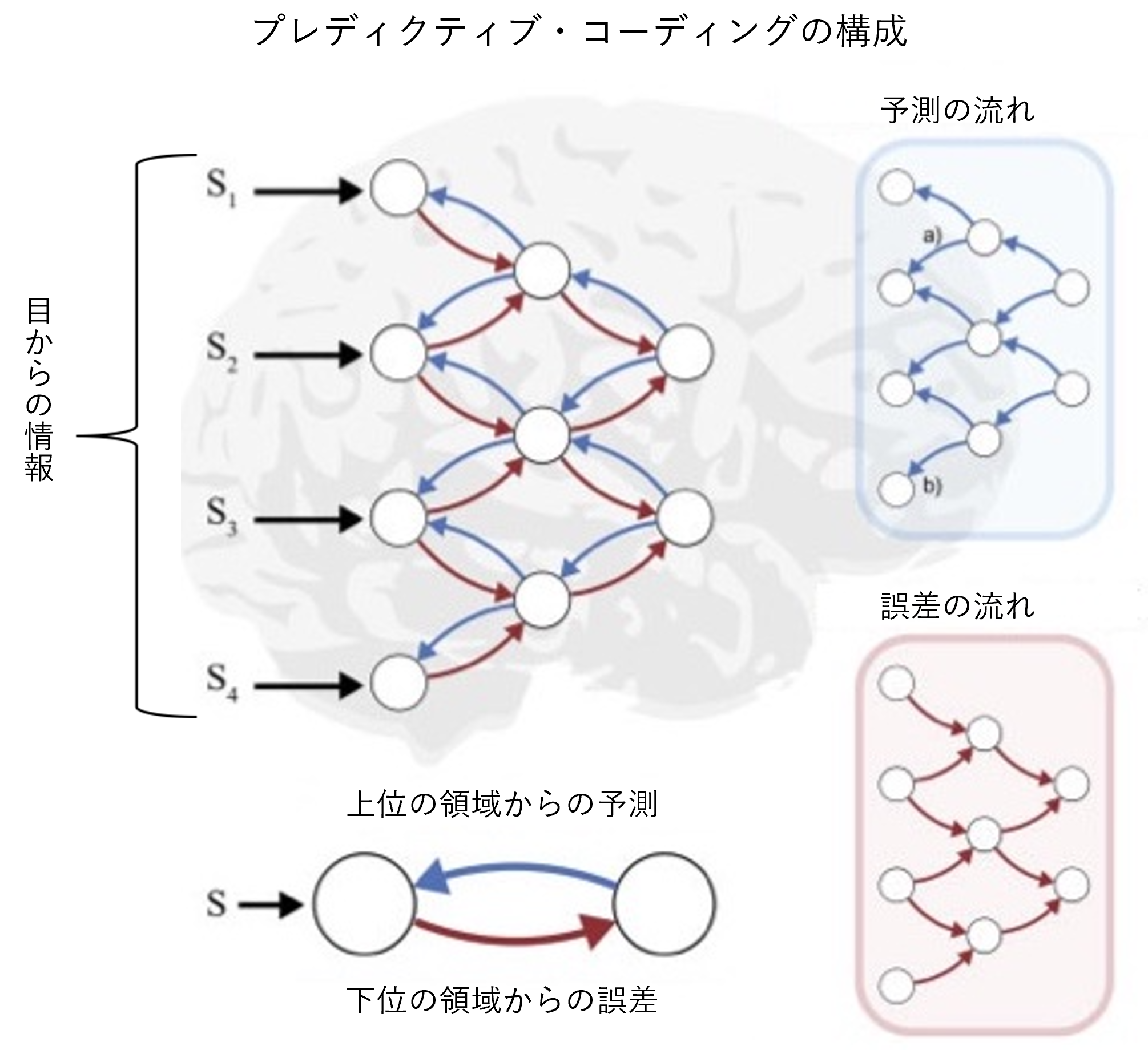
Successful integration of a world model into a robot
Based on these hypotheses, ARAYA is developing technologies to build and use world models by applying vae and predictive coding. Because of the nature of the basic research, which requires time to be put to practical use, we are working within the framework of projects promoted by the Japan science and technology agency (JST) under the ministry of education, culture, sports, science and technology (MEXT) and the new energy and industrial technology development organization (NEDO) under the ministry of economy, trade and industry (METI), with government budget support.
For example, as part of JST's Core Research for Evolutional Science and Technology (CREST) project, we have been aiming to develop artificial intelligence that gives the user, a human being, the feeling of being "conscious " (*5). The results of this work have culminated in a simple demonstration in March 2021. This system uses a camera-equipped robot to help people find items they have misplaced. Upon receiving a query from the user, the AI can answer the location of the object if it knows where it is, or send the robot to look for it if it does not.
5: Ryota Kanai, Construction of an intelligent information processing system for creative collaboration in harmony with humans, FY2019 Performance Report, https://www.jst.go.jp/kisoken/crest/evaluation/nenpou/r01/JST_1111083_15656376_ 2019_PYR.pdf
A feature of this system is that the robot follows its "curiosity" to choose the parts to look for. In the GQN system described above, it is possible to express numerically which parts of the world model are certain and which parts are not. This robot has a similar mechanism: it does not pay attention to the certain parts of the world model, but focuses on the uncertain parts. In other words, it has a pseudo-curiosity, a fascination with the unfamiliar. This allows us to find what we are looking for more efficiently.
Practical application for specific tasks first, aiming for "human level" in the end
The ultimate goal of general-purpose AI is a long way off. At present, the world models that GQN can acquire are limited to CG environments, and cannot represent the dynamics of the environment in relation to behaviour. Recently, there has been some research on acquiring spatial and temporal models of the world, and using these models to teach robots how to behave (policies), but these are usually based on the simple world created by CG (*6). (*6) Alaya's demonstration robot can only grasp a very limited range of environments, far less than the wide range of human capabilities.
6: See for example D. Ha et al., "World Models," https://arxiv.org/abs/1803.10122
However, we do not have to wait for practical applications to catch up with the human level. For specific tasks, AI with embedded world models may be available in the near future. Testing its usefulness in such small-scale applications can lead to a decision to move on to 'human-level' efforts, which require substantial development costs.
Alaya's initial application is for drones to be used to inspect power lines, for example, and to improve the efficiency of these inspections by choosing which areas to focus on according to their own curiosity. By applying the robotic technology described above, we believe that this will be possible in the not-too-distant future.
Click here to read more
Realization of general-purpose AI with the function of "consciousness" (1): Overview and three hypotheses
Realisation of general-purpose AI with 'consciousness' functions (2): Information generation theory - 'imagining' imaginary situations (this article)
Realization of general-purpose AI with the function of "consciousness" (3): Global workspace theory - Bridging information between brain modules (next article)
Realization of general-purpose AI with the function of "consciousness" (4): Meta-representation theory of qualia - Being able to cope with new tasks