REALISATION OF AGI WITH ‘CONSCIOUSNESS’ CAPABILITIES (3):
GLOBAL WORKSPACE THEORY – Bridging Information between Brain Modules
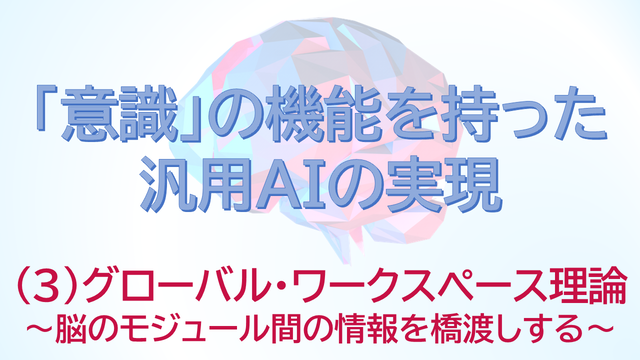
What kind of "consciousness" does a human being have - at ARAYA, we are continuing our research to answer this question. By creating an artificial equivalent of consciousness (AI), we are trying to get to the bottom of it and realize a "general-purpose AI".
In a four-part series, Ryota Kanai, President of the company, will explain how he plans to approach the project in concrete terms.
Realization of general-purpose AI with the function of "consciousness" (1): Overview and three hypotheses
Realization of general-purpose AI with the function of "consciousness" (2): Information generation theory - "imagining" an imaginary situation
Realization of general-purpose AI with the function of "consciousness" (3): Global workspace theory - bridging information between brain modules (this article )
Realization of general-purpose AI with the function of "consciousness" (4): Meta-representation theory of qualia - Being able to cope with new tasks
Bridging information between brain modules in a 'global workspace'
The function of consciousness we envisage is not only the ability to imagine imaginary situations using a world model. The second function of consciousness that could be useful in realising general-purpose AI is the ability to connect multiple functions in the brain through a common subconscious space. This is based on a hypothesis called the 'global workspace theory ', which explains the role of consciousness.
The Global Workspace Theory posits that the human brain is made up of a number of modules, each specialising in a particular function, such as sight, hearing, movement or language. Consciousness acts as a bridge between these modules. We believe that we are able to act appropriately in the real world because our consciousness is a clever coordination of several dedicated modules. The "global workspace" is the place where the information between the different modules is exchanged.
The global workspace theory was first proposed in the 1980s and has been supported by a number of experimental results (*1). Through computer simulations and other means, research has been carried out to determine which neural circuits shape the global workspace (*2). The concept of Consciousness Prior, developed by Professor Benzio of the University of Montreal, is also based on the global workspace theory (*3).
1: B. J. Baars, "The conscious access hypothesis: origins and recent evidence," TRENDS in Cognitive Sciences, Vol. 6, No. 1, January 2002.
2: G. A. Mashour et al., "Conscious Processing and the Global Neuronal Workspace Hypothesis," Neuron, 105, March 4, 2020.
3: Y. Bengio, "The Consciousness Prior," https://arxiv.org/abs/1709.08568
AI USING THE GLOBAL WORKSPACE IN COMBINATION WITH DEEP LEARNING
Following on from these earlier studies, Alaya is working on the development of AI technology for the global workspace. In December 2020, we published a paper describing our vision, co-authored by myself (Kanai) and the research director of the French research institute CerCo (Centre de Recherche Cerveau & Cognition) (*4). As described in the paper, we believe that the elemental technologies necessary for realisation already exist in individual cases.
*4: R. VanRullen et al., "Deep Learning and the Global Workspace Theory," https://arxiv.org/abs/2012.10390
The basic idea is to implement modules for a specific function in neural networks trained by deep learning, with a global workspace as the connecting mechanism between them. Deep learning is achieving human-like capabilities in many areas, such as image recognition, speech recognition, and language processing, so it's a natural idea to take advantage of this. the more neural networks AI can combine, the closer it gets to human-like versatility.
The question is how to share the information between the different neural networks. For example, if the visual neural network perceives a scene in front of it, the auditory neural network needs to be able to estimate which object is the sound captured by the auditory neural network, and the control neural network needs to be able to decide which action to take next. It is necessary to convey the information from the visual neural network in a form that can be used by other neural networks. In general, however, the inputs and outputs of neural networks are disparate for different purposes and are not always suitable for sharing information.
Information sharing between different neural networks is achieved by translating latent variables to each other
Our answer to the question of how to share information between different neural networks is a mechanism that translates the latent variables specific to each neural network into each other.
Neural networks that recognise images or speech reduce individual data containing vast amounts of information to the values of a small number of (low-dimensional) latent variables that are condensed from their features. The latent variables, packed with features of the original data, hold enough information to distinguish between different data. It is thanks to the latent variables that neural networks are able to classify images and distinguish speech properly.
However, there is no interchangeability between the latent variables used by the different neural networks. The idea is to implement the conversion mechanism between the latent variables as a global workspace.
There are already "seeds" of technology to transform latent variables. One example is a neural network that takes an image as input and outputs a sentence describing the content. In other words, it "translates" the image into text. These technologies have been developed on the basis of neural networks used for machine translation, and generally consist of an encoder that converts the image into latent variables, and a decoder that uses the latent variables to generate the text (*5). If this neural network is trained with a large amount of data consisting of pairs of images and sentences, it will be able to describe the scene captured by the camera as if it were written by a person. In a similar way, it appears theoretically possible to transform the latent variables of any neural network into the latent variables of another neural network.
5: S. Li et al., "Visual to Text: Survey of Image and Video Captioning," IEEE Transactions on Emerging Topics in Computational Intelligence, January 2019.
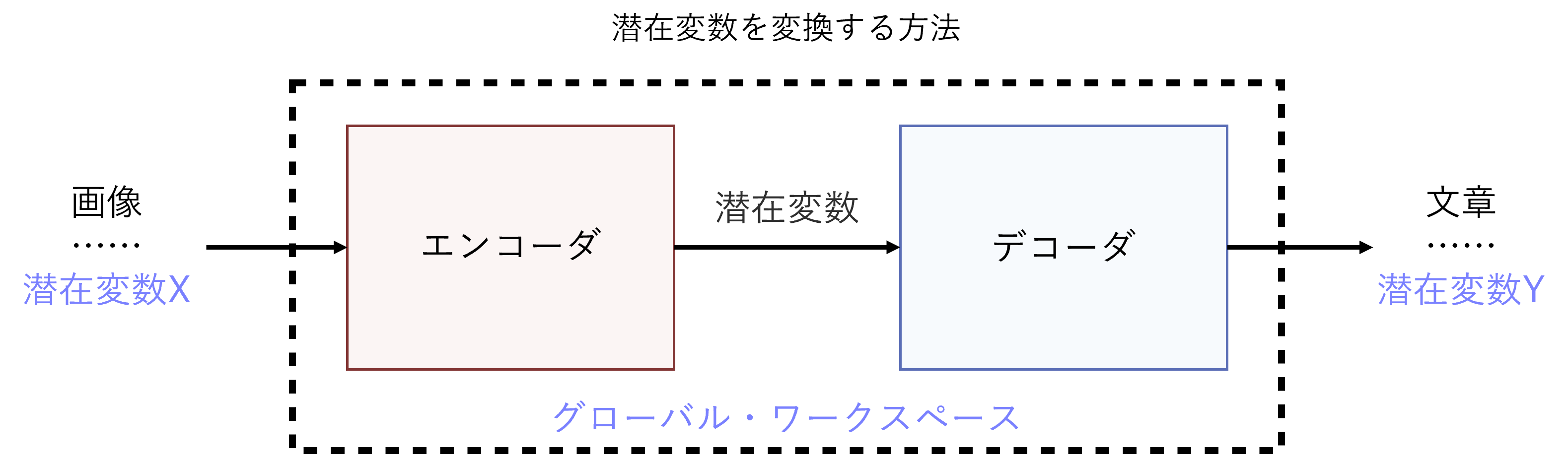
The aforementioned paper published in December 2020 also proposes a means by which a neural network, corresponding to a global workspace, can learn how to transform latent variables on its own. We envisage the use of so-called 'unsupervised learning', where the network can learn without a person providing the correct answer.
The criterion we rely on for learning is a principle we call 'cycle consistency'. For example, if a sentence in language A is translated into another language B and then translated back into the original language A, the neural network will be trained based on whether or not it returns to the same sentence. Previous research has shown that this method can be used to develop highly accurate neural networks for translation (*6). It is likely that similar methods can be used for translation between different latent variables.
6: G. Lample et al., "Unsupervised Machine Translation Using Monolingual Corpora Only," Available at: https://arxiv.org/abs/1711.00043
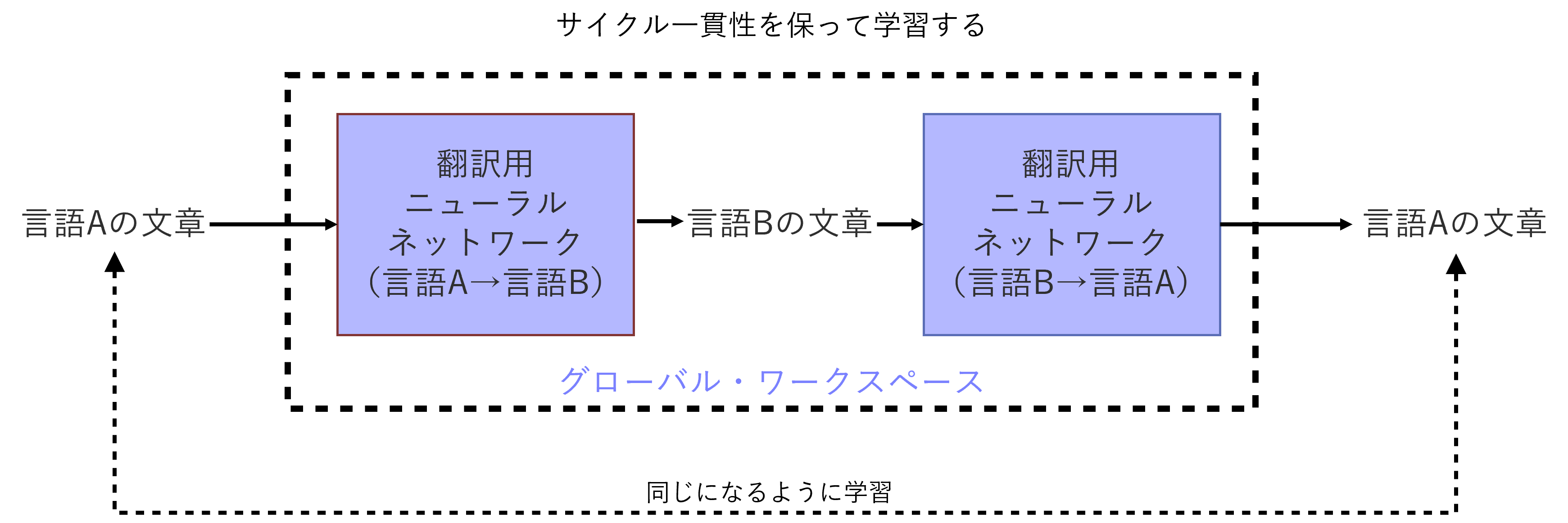
How the global workspace works
Based on these ideas, the aforementioned paper draws a blueprint of what a global workspace should look like and how it should work. The global workspace will be connected to a number of neural networks, including modules for recognising the external environment, such as vision and hearing, and modules for generating active behaviour, such as speech and movement. The Global Workspace assumes the ability to transform and communicate the values of latent variables for all of these combinations to each other.
Now suppose that an AI-powered robot with this configuration is searching for an object in a room. the global workspace is informed of the ever-changing object in a room. The global workspace is informed of the ever-changing situation in the room by the modules responsible for vision and hearing. The global workspace converts this information into values for latent variables in all the modules of the AI, which then pass the information on to, for example, the locomotion module, allowing it to explore the room. On the other hand, if the robot does not need to describe the situation in the room aloud, the speech module can be separated from the global workspace and no information will be sent to it.
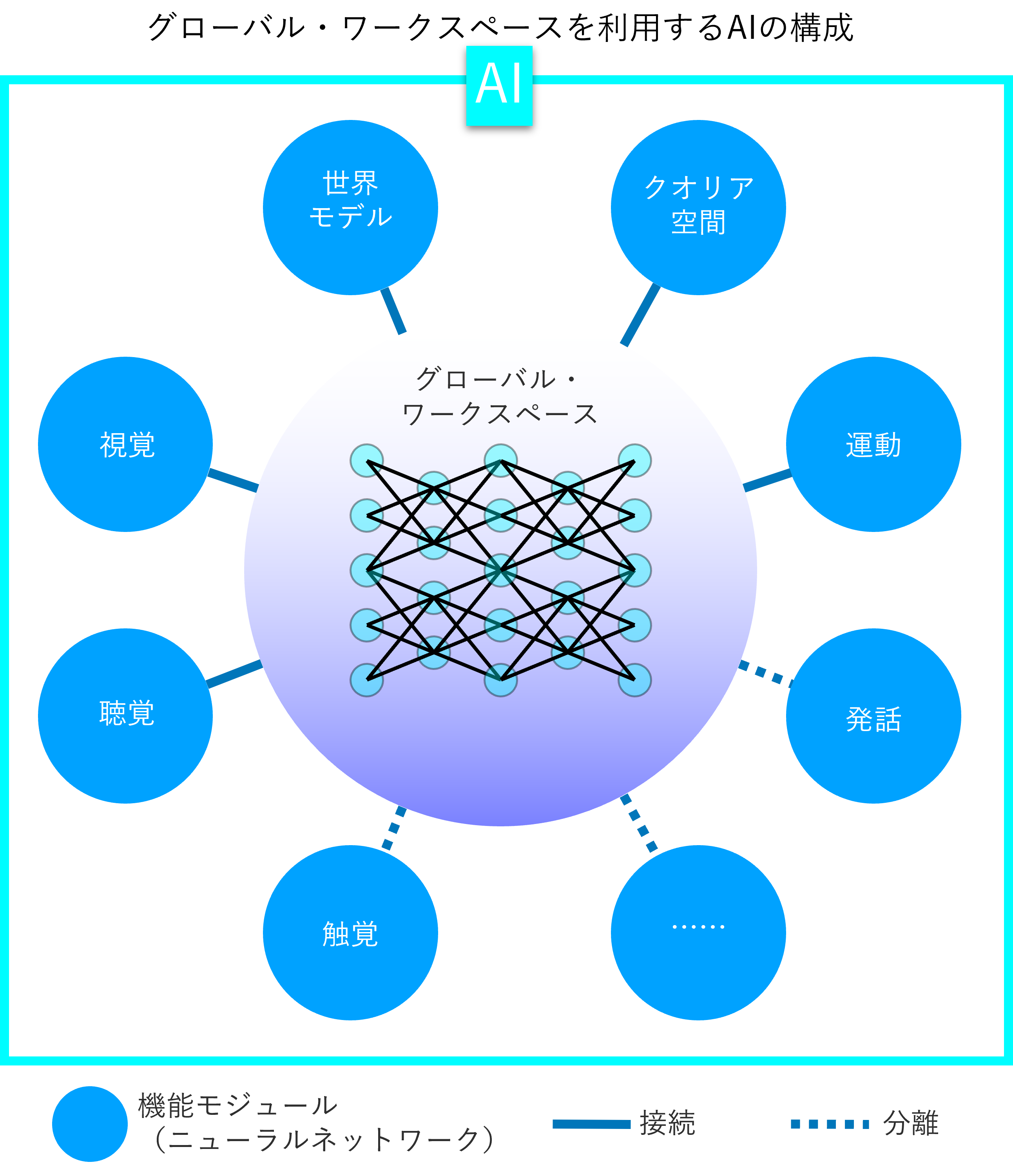
The information produced by each module at any given time does not always reach the Global Workspace. The latent variables, or 'contents of consciousness', that the Global Workspace takes up and transforms and transmits to other modules are determined top-down by the task currently being performed. The global workspace has a latent representation of the task itself, and the information in the module that matches this is selected.
We believe that we can use the so-called "attention" mechanism for this matching. Attention is a neural network mechanism used in machine translation to effectively incorporate information that is relevant to what is being processed.
In some cases, the information that the global workspace takes up and sends to each module is determined bottom-up, depending on the signals from the modules. For example, a child might suddenly jump out in front of the robot while it is searching for something. This kind of urgent information is immediately uploaded to the global workspace, and the motion module takes immediate action, such as triggering an evasive action. A speech module can also be connected to the workspace at a moment's notice to say "Look out! is also possible.
The implementation of the Global Workspace is also related to the generation of information based on the World Model, which we have identified as the primary function of consciousness. Simply put, the world model can be positioned as one of the modules leading to the global workspace. It is a counterfactual simulation of the world-model that comes to consciousness as information in the workspace.
Click here to read more
REALISATION OF AGI WITH ‘CONSCIOUSNESS’ CAPABILITIES (1):Overview and Three Hypotheses
REALISATION OF AGI WITH ‘CONSCIOUSNESS’ CAPABILITIES(2):INFORMATION GENERATION THEORY – ‘Imaging’ Imaginary Situations
REALISATION OF AGI WITH ‘CONSCIOUSNESS’ CAPABILITIES (3):GLOBAL WORKSPACE THEORY – Bridging Information between Brain Modules
REALISATION OF AGI WITH ‘CONSCIOUSNESS’ CAPABILITIES (4):META-REPRESENTATION THEORY OF QUALIA – Being Able to Cope with New Tasks